Contributors
Faculty Mentor: C. Dinitra White
Preview
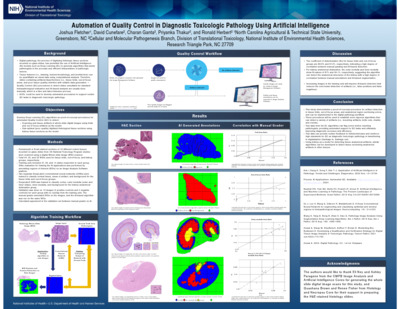
Description
Poster presented at Research Experiences for Undergraduates, North Carolina Agricultural and Technical State University, Greensboro, NC
This poster explores the role of Artificial Intelligence (AI) and Deep Learning (DL) in digital pathology, specifically in the process of digitizing histologic tissue sections mounted on glass slides. The use of AI models has enhanced the ability of pathologists to interpret pathologic lesions with greater accuracy and efficiency. Tissue features, such as staining, lesions, morphology, and architecture, can be quantified as visual data through computational analysis. However, the presence of artifacts or imperfections—such as tissue folds, out-of-focus areas, and poor tissue quality—can compromise the reliability of data generation. Currently, Quality Control (QC) procedures to identify unsuitable slides for histopathological evaluation and AI analysis are performed manually, which is both time-consuming and labor-intensive. This research suggests that AI and DL technologies could be leveraged to automate these QC tasks, streamlining diagnostic toxicologic pathology workflows.
Format
PNG
Keywords
digital pathology, artificial intelligence, deep learning, histologic tissue sections, pathologic lesions, computational analysis, tissue features, staining, morphology, architecture, quality control, slide imperfections, tissue folds, out-of-focus areas, poor tissue quality, diagnostic toxicologic pathology, automated procedures, QC tasks, AI-based analysis